Supervised Ph.D. Students
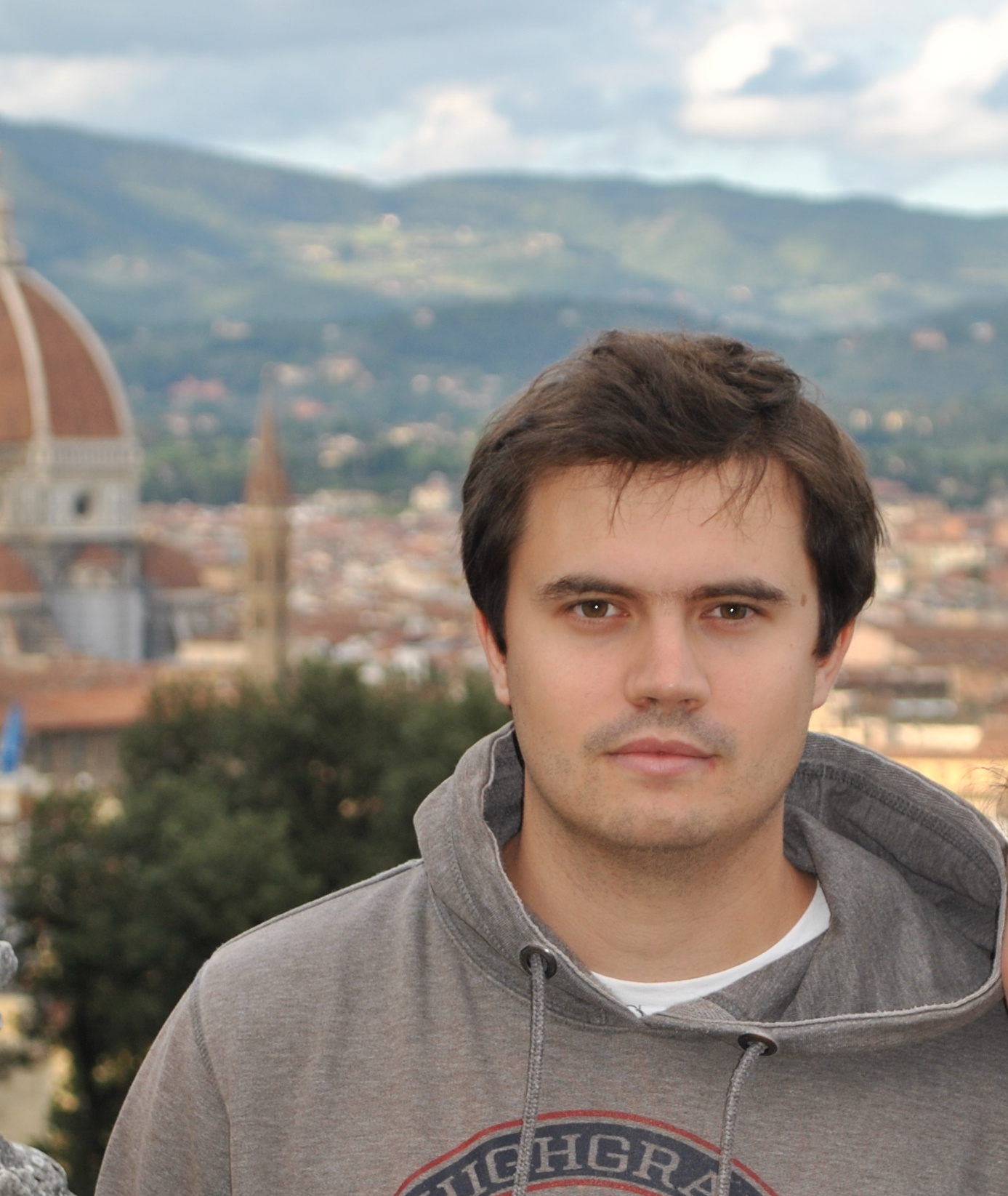
Maxime Devanne
February 2012 - December 2015.
Supervised in collaboration with Mohamed Daoudi and Pietro Pala
Thesis title: 3D Human Behavior Understanding by Shape
Analysis of Human Motion and Pose.
Abstract: The emergence of RGB-D sensors providing the 3D structure of both the scene and the human body offers new opportunities for studying human motion and understanding human behaviors. However, the design and development of models for behavior recognition that are both accurate and efficient is a challenging task due to the variability of the human pose, the complexity of human motion and possible interactions with the environment. In this thesis, we address this issue in two main phases by differentiating behaviors according to their complexity. We first focus on the action recognition problem by representing human action as the trajec- tory of 3D coordinates of human body joints over the time, thus capturing simultaneously the body shape and the dynamics of the motion. The ac- tion recognition problem is then formulated as the problem of computing the similarity between shape of trajectories in a Riemannian framework. Experiments carried out on four representative benchmarks demonstrate the potential of the proposed solution in terms of accuracy/latency for a low-latency action recognition. Second, we extend the study to activities by analyzing the evolution of the human pose shape to decompose the motion stream into short motion units. Each motion unit is then character- ized by the motion trajectory and depth appearance around hand joints, so as to describe the human motion and interaction with objects. Finally, the sequence of temporal segments is modeled through a Dynamic Naive Bayesian Classifier. Experiments on four representative datasets evaluate the potential of the proposed approach in different contexts, including gesture or activity recognition and online activity detection
Presentation: PhD. Defense
Quentin De Smedt
October 2014 - December 2017.
Supervised in collaboration with Jean-Philippe Vandeborre
Thesis title: Dynamic hand gesture recognition - From traditional handcrafted to recent deep learning approaches.
Abstract: Hand gestures are the most natural and intuitive non-verbal communica- tion medium while using a computer, and related research efforts have recently boosted interest. Additionally, data provided by current com- mercial inexpensive depth cameras can be exploited in various gesture recognition based systems. The area of hand gesture analysis covers hand pose estimation and gesture recognition. Hand pose estimation is con- sidered to be more challenging than other human part estimation due to the small size of the hand, its greater complexity and its important self occlusions. Beside, the development of a precise hand gesture recognition system is also challenging due to high dissimilarities between gestures derived from ad-hoc, cultural and/or individual factors of users. First, we propose an original framework to represent hand gestures by using hand shape and motion descriptors computed on 3D hand skeletal fea- tures. We use a temporal pyramid to model the dynamic of gestures and a linear SVM to perform the classification. Additionally, we create the Dynamic Hand Gesture dataset containing 2800 sequences of 14 gesture types. Evaluation results show the promising way of using hand skele- tal data to perform hand gesture recognition. Experiments are carried out on three hand gesture datasets, containing a set of fine and coarse heterogeneous gestures. Furthermore, results of our approach in terms of latency demonstrated improvements for a low-latency hand gesture recog- nition systems, where an early classification is needed. Then, we extend the study of hand gesture analysis to online recognition. Using a deep learning approach, we employ a transfer learning strategy to learn hand posture and shape features from depth image dataset originally created for hand pose estimation. Second, we model the temporal variations of the hand poses and its shapes using a recurrent deep learning technology. Finally, both information are merged to perform accurate prior detection and recognition of hand gestures. Experiments on two datasets demon- strate that the proposed approach is capable to detect an occurring gesture and to recognize its type far before its end.

Théo Voillemin
Personalized augmented reality assistance by hand gesturerecognitionusing head-mounted display
October 2019 - September 2021.
Supervised in collaboration with Jean-Philippe Vandeborre
News
-
- We organize the international workshop UHA3DS 2016 hosted by ICPR 2016
- We organized the international workshop UHA3DS 2015 hosted by IEEE FG 2015
Dr. Rim Slama
November 2011 - October 2014.
Supervised in collaboration with Mohamed Daoudi
Thesis title: Geometrical Approach for 3D Human Motion Analysis: Application to Action Recognition and Retrieval.
Abstract: In this thesis, we focus on the development of adequate geometric frameworks in order to model and compare accurately human motion acquired from 3D sensors. In the first framework, we address the problem of pose/motion retrieval in full 3D reconstructed sequences. The human shape representation is formulated using Extremal Human Curve (EHC) descriptor extracted from the body surface. It allows efficient shape to shape comparison taking benefits from Riemannian geometry in the open curve shape space. As each human pose represented by this descriptor is viewed as a point in the shape space, we propose to model the motion sequence by a trajectory on this space. Dynamic Time Warping in the feature vector space is then used to compare different motions. In the second framework, we propose a solution for action and gesture recognition from both skeleton and depth data acquired by low cost cameras such as Microsoft Kinect. The action sequence is represented by a dynamical system whose observability matrix is characterized as an element of a Grassmann manifold. Thus, recognition problem is reformulated as a point classification on this manifold. Here, a new learning algorithm based on the notion of tangent spaces is proposed to improve recognition task. Performances of our approach on several benchmarks show high recognition accuracy with low latency.